“Our goal is to create an automated degree-planning system that would provide timely feedback to college students.”
— Huzefa Rangwala, professor in the Mason Engineering's Department of Computer Science
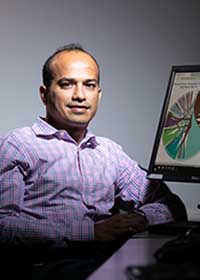
Huzefa Rangwala is a professor of computer science. Photo by Ron Aria.
College students may one day choose their courses the same way they choose books on Amazon. A computer program will suggest the best classes for them, based on grades in other courses, career goals, instructor preference, and learning style.
Huzefa Rangwala, professor in the Mason Engineering's Department of Computer Science, and his research team are using educational data-mining to build the foundation for personalized course-selection software to help undergraduates succeed in college. “Our goal is to create an automated degree-planning system that would provide timely feedback to college students.”
“We need to identify students at risk of dropping out and see if we can help them succeed,” Rangwala says. “Nationally, only about 59 percent of full-time college students at four-year institutions graduate in six years.”
Existing educational technologies assist students in choosing majors and selecting classes, but most don’t consider such factors as past academic performance, career goals, and capabilities, he says.
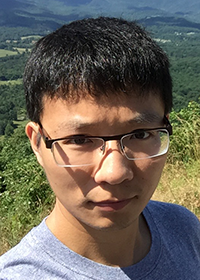
Qian Hu, a PhD student in computer science, is working on the educational data-mining research.
His research team analyzes college students’ academic history, including high school grade-point averages, SAT scores, and college grades, to develop new algorithms. These machine-learning models will drive degree-planning software to help students make more personalized course selections.
This research could lead to the creation of systems that could help students:
- Identify the best courses for their career goals.
- Pinpoint the best sequence of classes towards better learning and workforce readiness.
- Plan their schedules and workloads based on how many hours of study a week each course would require.
- Determine which topics they should review from previous classes to be successful in upcoming courses.
- Decide which instructors and courses are best suited for their learning style.
This “educational early-warning system” could help guide students at risk of failing some courses, increasing their chances of getting degrees, says Qian Hu, a doctoral student on the project.
“Our model may help predict the best combination of courses a student should take in the next semester,” adds Zhiyun Ren, a PhD student who works on the research.
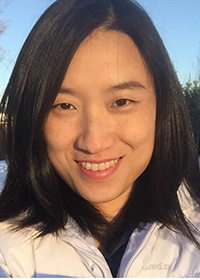
Doctoral student Zhiyun Ren says the research model may help predict the best combination of courses for a student.
Rangwala received $750,000 from the National Science Foundation for this work, which began in 2014. He is collaborating with Aditya Johri, associate professor in Mason Engineering’s Department of Information Sciences and Technology; Jaime Lester, a professor in Mason’s Higher Education Program; and researchers with the University of Minnesota.
Rangwala says the research wouldn’t have been possible without the help of Mason’s Offices of Institutional Research and Effectiveness, Admissions, University Life, Information Technology Services, Student Academic Affairs, and the Registrar.