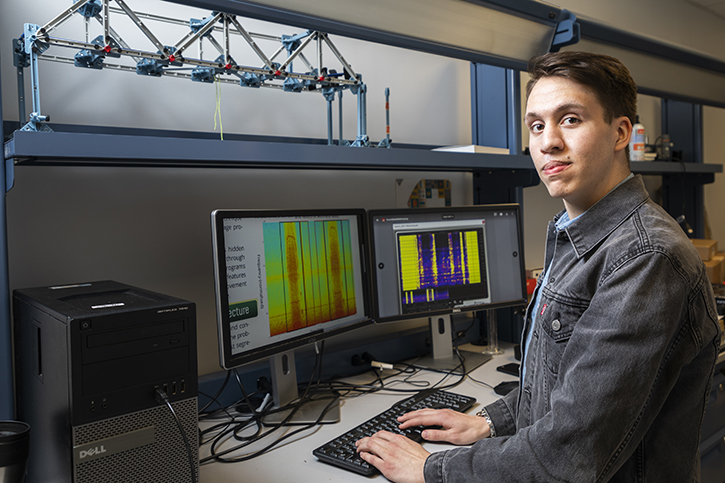
Gabe Earle, a rising senior majoring in civil and infrastructure engineering, is trying to develop what a professor called "a completely new approach for the monitoring of industrial equipment." Photo by Lathan Goumas.
Gabe Earle has a passion for both music and engineering.
The rising senior at George Mason University is a drummer in the university’s nationally recognized pep band, the Green Machine, and is majoring in civil and infrastructure engineering.
A unique research opportunity, through Mason’s Office of Student Scholarship, Creative Activities and Research (OSCAR), allowed him to combine both those interests as he examined the feasibility of using acoustic data signals to inspect and analyze the movements of manufacturing infrastructure.
According to Volgenau School of Engineering assistant professor David Lattanzi, who helped oversee Earle’s research with master’s student Jeff Bynum, it is “a completely new approach for the monitoring of industrial equipment.”
“What we did was develop a convolutional neural network [CNN] to input the audio data and output an explanation of what the machine is doing,” Earle said. “We analyzed audio recordings of those machines for irregularities.”
The network, a deep learning algorithm that can assign importance to various aspects of an image, is essentially an artificial intelligence, Earle said.
The network does its evaluations through image-like spectrograms that extract features that represent the acoustic signature of specific machine movements. Through repetition, the network detects configurations that deviate from a baseline “training set.”
In practice, anomalies detected will alert managers that maintenance is necessary to prevent damage and a loss of efficiency.
Lattanzi called Earle “the gold standard for an undergraduate researcher” because of his curiosity, enthusiasm and insight.
Earle’s project began when a tech company asked Mason’s Volgenau School of Engineering for an innovative solution to improve monitoring at its industrial facility. With several years of experience in audio recording and analysis, earned by producing his own music, Earle had the ideal background to take on the project.
“Music and engineering are not usually related in people’s minds,” Earle said. “Even I used to keep my hobby separate from school. But the research instrumentation ended up being so similar to the recording gear I was used to, and I had already unknowingly studied a lot of methodology such as signal processing through my producing hobby. So this project was the a great introduction to working on the bleeding edge of acoustics in civil engineering.”
Earle said the CNN was able to isolate critical machine activities 87 percent of the time, and it was able to recognize and analyze the most important machine motions more than 93 percent of the time. This accuracy demonstrated, he said, that acoustic-monitoring data hold great promise for a nonintrusive method of industrial monitoring.
And that kind of advanced data analytics, Lattanzi said, has value for any organization that needs to maintain industrial systems. It offers a new way of monitoring systems, such as construction equipment, ships, and smart infrastructure systems, just through the use of a well-placed microphone.